Since ChatGPT became public, there has been an enormous buzz around AI and AI-generated content. People started chatting with AI for fun and quickly began thinking about how AI could help their daily work. The product management industry also took note, adding more and more AI features to its solutions. Today, AI has become a crucial part of many products, leading to the rise of AI product management tools.
However, there are still risks associated with using AI. AI can be beneficial in some areas, while in others, it cannot replace human logic and thinking. Follow us in this article to learn how to use AI in product management effectively and where it is essential to maintain the human factor.
The rise of AI among product management tools
AI-powered tools are transforming every stage of the product management lifecycle. AI can analyze vast datasets during market research to identify emerging trends and consumer behaviors, offering a competitive edge. In product development, AI assists in feature prioritization by predicting user needs and simulating various product scenarios, thus saving time and resources.
Additionally, AI-driven customer support tools like chatbots provide real-time assistance, enhancing customer satisfaction and freeing human resources for more complex tasks. The rise of AI among product management tools marks a new era where data-driven insights and automation play a crucial role in driving product success.
When AI is Good for Product Management
Market Research
Read and highlight pain points in customer feedback: AI can sift through large volumes of customer reviews, comments, and feedback to identify common issues and complaints. This helps product managers quickly pinpoint areas needing improvement without manually reviewing all the data. For instance, natural language processing (NLP) algorithms can detect recurring phrases or sentiments that indicate dissatisfaction, enabling a more efficient response to customer needs.
Provide an overview of a market (advantageous when the product team is new to the industry): AI can analyze market data from various sources to comprehensively understand the industry landscape. This includes identifying key competitors, market size, growth trends, and emerging opportunities. AI can accelerate the learning curve for product teams new to a particular industry by providing detailed insights and analytics that would otherwise take much longer to gather and interpret manually.
Tools: ChatGPT
Doing the User personas research
AI is highly effective in conducting user personas research, a crucial step in understanding target customers. AI can identify patterns and trends in user behavior by analyzing large datasets from various sources, such as social media, customer reviews, and web analytics. This allows product managers to create detailed and accurate user personas without the manual effort traditionally required.
AI can segment users based on their behavior, preferences, demographics, and other relevant factors. It can highlight common characteristics among different user groups, providing a deeper understanding of their needs, motivations, and pain points. By doing so, AI helps product managers develop more targeted and personalized product development and marketing strategies. The speed and accuracy of AI in processing vast amounts of data make it an invaluable tool for creating user personas that genuinely reflect the diversity and complexity of the customer base.
Tools: ChatGPT, StoriesOnBoard AI
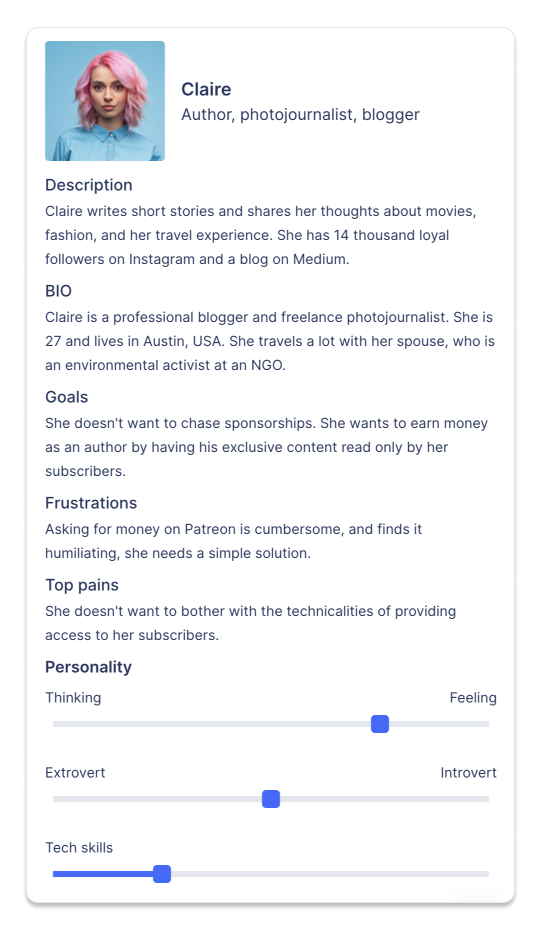
StoriesOnBoard allows you to create user personas with extensive details. Adding these user personas to the product backlog helps achieve a shared understanding of the target user and enables the development of features that meet their specific needs.
Writing repeating or common backlog items
Kickstarting a new product often involves repetitive tasks where innovation isn’t necessary. Examples include signup processes, integration steps, checkout screens, subscription pages, and user profile management. These common interfaces are well-known to AI, so don’t hesitate to ask for help.
In addition to repetitive tasks, some features are straightforward for AI to suggest because they are available on competitors’ websites. Adding these popular and valuable features to your backlog can serve multiple purposes:
- Ensuring you don’t forget them
- Providing a solid foundation to improve upon features that competitors already offer
- Sparking new ideas on how to enhance or provide better solutions for existing features
StoriesOnBoard AI lets you jumpstart your project by collecting the first few user stories. These stories are organized under the product backbone on a user story map, making it easy to extend with new directions or dive deeper into specific features.
Tools: StoriesOnBoard AI
Brainstorming niche ideas
Product managers can use AI to generate a list of potential ideas and decide which ones to pursue. AI can provide a fresh perspective and uncover opportunities that might have been overlooked. Additionally, if the initial suggestions are unsatisfactory, product managers can ask AI for more recommendations, tailoring the inputs to refine and improve the output. This collaborative approach allows human creativity and judgment to work with AI’s data-driven insights, leading to more innovative and well-rounded product strategies.
To do that, you should always input existing features and provide details about the target persona. Once you have collected these inputs, try to ask for “niche” or “uncommon” user stories, or you can create your own direction. For example, you could ask for “features that allow purchasing food online without needing a bank card.”
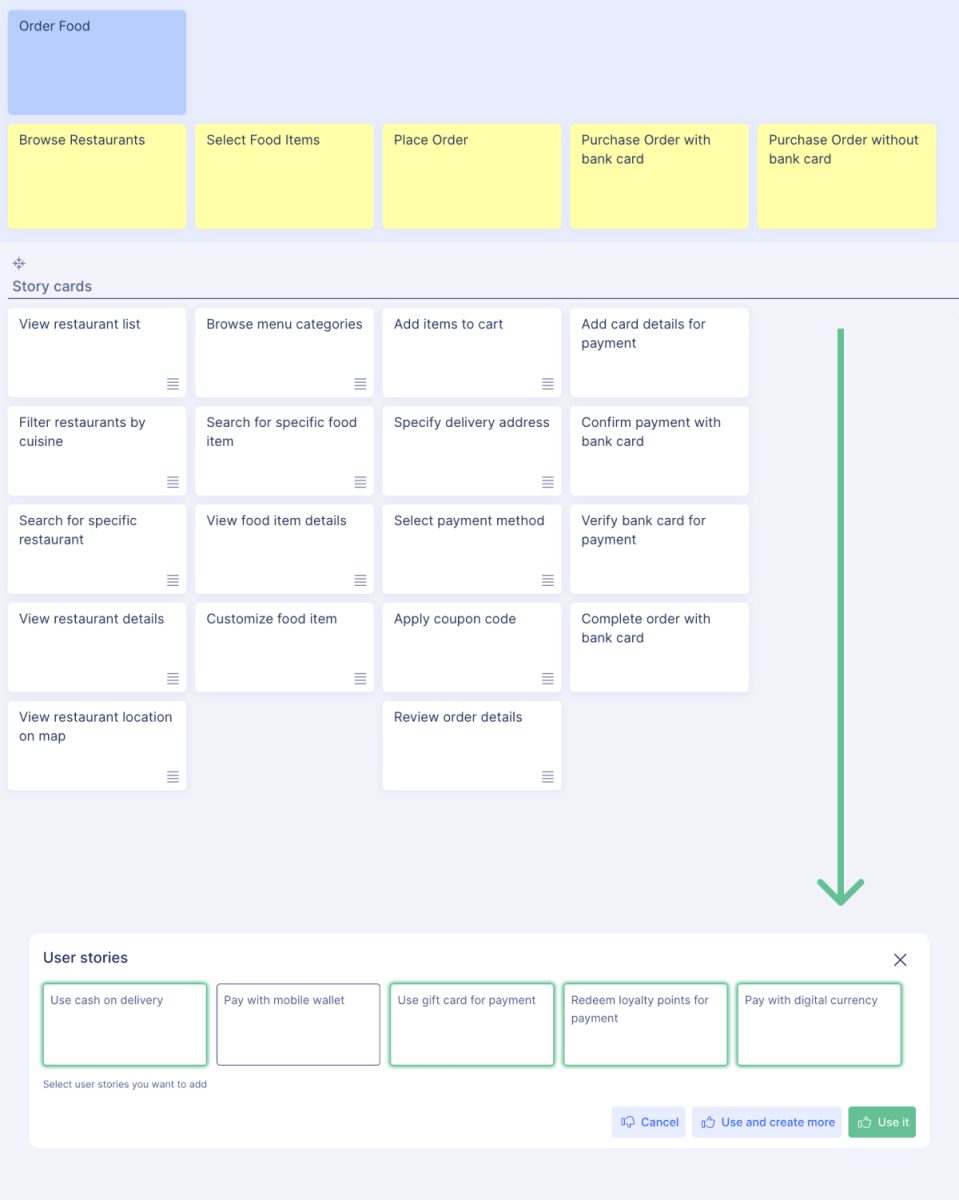
You can enter these inputs into the ChatGPT interface or add new stories directly into StoriesOnBoard. A significant benefit of using StoriesOnBoard AI is that it can read your backlog and avoid redundancy among the user stories. While StoriesOnBoard AI doesn’t provide a chat-like interface, you should create a top-level card manually and then ask the AI to brainstorm user stories.
Tools: ChatGPT, StoriesOnBoard AI
When AI Might Fall Short
Setting up priorities
AI can struggle to establish priorities in product management. While it can analyze data and suggest potential priorities based on patterns and algorithms, it often lacks the nuanced understanding required to make strategic decisions. Priorities in product management can shift rapidly due to various factors, such as customer requests, client requirements, market situations, or changes in brand and product strategy.
For instance, an urgent customer need or a sudden market trend might require immediate attention, which AI might not accurately account for without real-time contextual understanding. Additionally, brand strategy often involves long-term goals and subjective judgment calls that AI cannot fully grasp. Human product managers are essential in evaluating these dynamic factors and making informed decisions aligning with immediate needs and long-term objectives.
However, AI can still provide valuable insights as another team member. It can analyze large volumes of data quickly, highlight trends, and suggest possible priorities based on historical data and predictive models. These insights can serve as a helpful starting point, allowing human product managers to make more informed decisions. Product managers can set more effective and responsive priorities by combining AI-generated insights with human expertise.
How to make it less painful?
StoriesOnBoard simplifies the process of setting priorities among user stories. You can collaboratively score the items with the client, making it a straightforward task. User story maps are designed to be easily understood, even for non-technical stakeholders. You can confidently invite teammates from other departments or clients to participate in the prioritization session. Additionally, you can choose from pre-made prioritization templates like MoSCoW and KANO to streamline the process further.
Estimating backlog items
AI faces significant challenges when it comes to accurately estimating backlog items. Estimating the effort, time, and resources required for various tasks in a product development backlog involves a deep understanding of the project’s context, team dynamics, and potential obstacles. While AI can analyze historical data and suggest estimates based on past performance, it often falls short in accounting for the unique aspects of each new task.
Estimations can vary widely based on factors such as the feature’s complexity, the team’s expertise, unforeseen technical challenges, and shifting project requirements. AI might not fully grasp these nuances, leading to either overly optimistic or too conservative estimates. For example, a task that appears straightforward based on past data might involve hidden complexities that only experienced team members can foresee.
However, AI can still contribute valuable insights as a part of the team. It can provide initial estimates based on data-driven analysis, serving as a baseline for further refinement. Human input is crucial to adjusting these estimates, considering the specific context and any new information. Product managers can achieve more accurate and realistic backlog estimations by leveraging AI’s analytical capabilities alongside human judgment and experience.
How to make it less painful?
You have two options for setting up estimations in StoriesOnBoard. You can start with the rough estimation by marking user stories using T-shirt sizes and adding story points or hours.
Handling New Market Conditions
AI might struggle with new, unique market conditions where historical data isn’t available. In such scenarios, the lack of past data makes it challenging for AI to provide accurate predictions or insights. Human intuition and experience become crucial in these situations, as they can interpret subtle market signals and adapt to rapidly changing environments.
Product managers must leverage their expertise to navigate these uncertainties, making informed decisions based on their industry knowledge, customer behavior, and emerging trends. Combining AI’s data processing capabilities with human intuition can lead to more robust and adaptive strategies in these novel market conditions.
Dealing with Complex Customer Queries
AI may not handle complex customer queries well, leading to frustration. While AI chatbots and automated support systems can efficiently manage routine inquiries and provide quick responses, they often fall short when faced with intricate or nuanced issues.
These limitations can result in customer dissatisfaction and a sense of being misunderstood. Human support is still needed for complex issues, as trained professionals can offer personalized assistance, empathize with customer concerns, and provide creative solutions that AI might miss. Integrating AI with human support ensures a balanced approach, where AI handles more straightforward tasks, freeing human agents to focus on more challenging and critical customer needs. This synergy enhances overall customer satisfaction and maintains a high standard of service.
Summary
AI has revolutionized product management by enhancing efficiency and decision-making across various stages. Tools like ChatGPT and StoriesOnBoard AI can analyze vast datasets, identify market trends, and understand customer behaviors, making them invaluable for market research. They also help create detailed user personas and manage repetitive tasks such as signup processes and feature prioritization, allowing product managers to focus on more strategic aspects of product development.
However, AI has its limitations. It struggles with new, unique market conditions where historical data is unavailable, making human intuition and expertise essential for interpreting subtle market signals and adapting to changes. AI also falls short in handling complex customer queries, where personalized assistance from human agents is necessary to provide satisfactory solutions. Thus, while AI can handle routine tasks and provide data-driven insights, the human factor remains crucial for nuanced decision-making and addressing complex issues, ensuring a balanced and effective approach to product management.